The Shortcomings of Artificial Intelligence
A Comprehensive Study
Stevie A. Burke, Ammara Akhtar
Affiliation: Clean Community Inca , North Carolina, United States
Corresponding author: Stevie A. Burke
Email Ids : Stevie@cleancommunityinc.com, Ammaraakhtar3@gmail.com
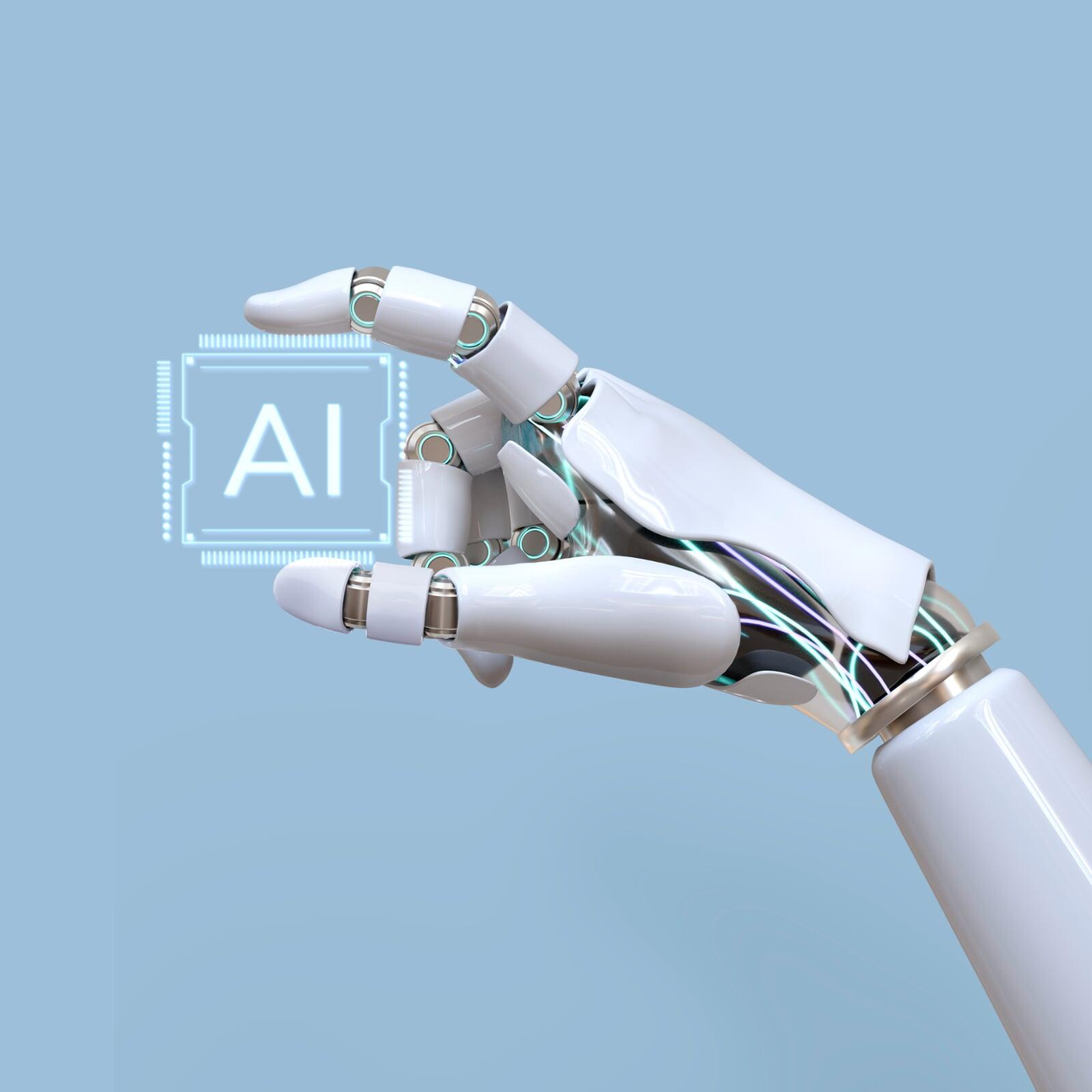
Abstract
Keywords: AI, artificial intelligence, Robots, Chat-GPT, Machine learning.
Introduction
Artificial intelligence is considered one of the highly disruptive innovations of the 21st century that has garnered the enormous attention of the global community. Artificial Intelligence (AI) provides unprecedented widespread opportunities for revolutionizing and upgrading the infrastructure of various industries. It is one of the faster-growing go-to technology for global industries allowing personalized experiences for all individuals. This disruptive technology is evolving and getting smarter daily, offering unique and incredible applications, including robots, facial recognition, customized user experience, language comprehension, voice assistant, and autonomous vehicles. In this study, various challenges regarding implementing AI in diverse fields of human lives were discussed. Indeed, AI is a long-standing controversial topic and is sometimes illustrated negatively. In an ongoing debate, some would refer to it as a blessing in disguise for businesses and industries. In contrast, others would refer the artificial intelligence technology as an endangerment to the existence of humans due to its potential ability to take over and overpower humankind.
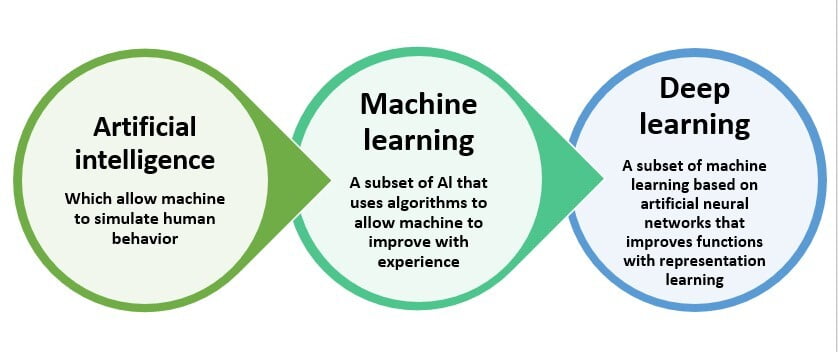
AI can alter the interaction between different businesses and their customers (increasing productivity and improving effectiveness), thereby maximizing business profit. Online companies adopt chatbots to offer their consumers full-time services (Luo et al., 2019). AI-based robots are employed to assist customers in selecting the right products. In the healthcare system, artificial intelligence-based diagnostic systems allow rapid medical imaging, improved treatment planning, and faster diagnostics (Ploug & Holm, 2020). In the transportation industry, the manufacturing of autonomous vehicles has grabbed massive attention from different sectors (Radhakrishnan & Chattopadhyay, 2020). Additionally, AI-based technologies, including deep learning, voice assistant, facial recognition (Xu et al., 2021), image processing, personalized learning, and language translation, are bringing convenience to various aspects of human lives (Bhutoria, 2022). Despite these advantages, AI can potentially induce numerous negative consequences at different individual and organizational levels (Alt, 2018; Cheng, (2022)). Currently, the positive features of AI are receiving massive attention, while little is paid to the negative ones.
Considering the availability of limited research on the downsides of artificial intelligence, this study was conducted to provide insight into the dark realm of AI and encourage the researchers to study and explore the risks associated with using artificial intelligence. AI undoubtedly has the potential to bring technological innovation in numerous contexts, but we should not overlook the detrimental consequences associated with using AI (Floridi et al., 2021).
The shortcomings of AI
Artificial intelligence is omnipresent, and the belief of it becoming hostile against us is not new, as history is full of warnings ranging from famous sayings like the one by Elon Musk that "AI is far more dangerous than nuclear weapons" (Castagno & Khalifa, 2020). Stephen Hawking's said that "the progressive development of complete AI could fully demolish the human race as this disruptive technology has the potential to fully re-design itself at a constantly increasing rate, while humans that exhibit steady biological evolution rate would not be able to compete and therefore could be potentially superseded" to the bulk of movies signifying the danger of AI turning from a faithful companion to an evil enemy (Curchoe & Bormann, 2019). Artificial intelligence has different cons, including high costs to create machines that mimic humans, inability to think outside the box (lacking creativity), displacing human occupations with robots, promoting human laziness, and the bulk of others. From the societal perspective, artificial intelligence is yielding various dark impacts ranging from data security to privacy issues, ethical problems, and workforce replacement (Dwivedi et al., 2021) (Figure 2).
Data base
AI has the potential to integrate the bulk of data and modify it in a way that leads to the development of selection tools, AI-based predicted models, and pattern recognition. But unfortunately, the AI-based science of "bulk data" employing computational strategies of big data sets to recognize unique patterns and connections also causes significant challenges ranging from data bias, data accessibility problems, data security, and ownership issues. The algorithms used in ML go through a training phase and is dependent on sufficient data input. Any systematic errors or inexactness during the steps of DL can substantially cause bias amplification or the absolute disregard of specific kinds of data. For instance, in the healthcare field, if the data at the training stage only include embryos selected for transfer, the training dataset will not be representative of the whole embryos data on which it is supposed to be used later, thereby inducing data bias in the embryology laboratory (Curchoe & Bormann, 2019).
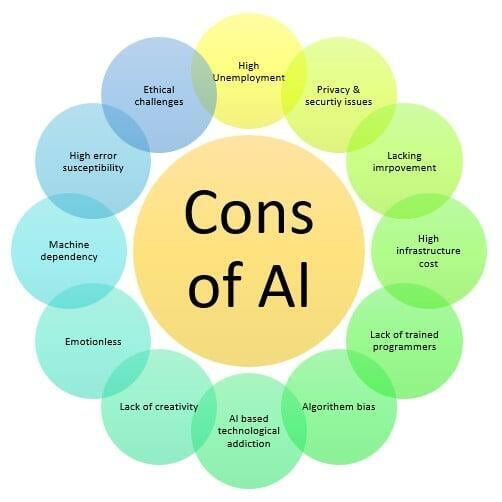
Figure 2: The cons of AI
AI carbon footprint
AI carbon footprint is another emerging debatable ethical concern of artificial intelligence technologies with a truthfully global dimension. The growing pervasiveness of artificial intelligence technologies has directed an evolving apprehension about the AI carbon footprint and its devastating impact on global warming. The emission of greenhouse gases (GHG) is stimulated by electricity and computational resources that are needed to train AI models through machine learning methods (Tamburrini, 2022). Strubell et al., (2019) studied and calculated the carbon footprint of training an AI-based model, "Natural language processing" (NLP). He reported that the training of this AI-based model could be estimated to emit greenhouse gases equal to those emitted by five automobile vehicles. Furthermore, it has been reported that the estimated carbon footprint varies according to the locations, the overall demand, and global differences in power sources. The training of the AI-based model "BERT" has been found to emit approximately 22-28 kg of carbon dioxide in the data center of the US, which is twice the amount of emission generated by performing a similar experiment in Norway (Gibney, 2022).
Failure of chatbots
AI technology has been widely employed as a problem-solving tool that provides high efficiency and increased effectiveness. Recently, a chatbot named Chatbot" ChatGPT has been gaining widespread attention from people around the world. ChatGPT is an AI-based chatbot developed with the latest version of OpenAI and launched at the end of 2022. Although there is much hype globally, in practice, like any other chatbot, these AI-based chatbots have raised several disadvantages, including lack of expression, no feelings, lack of insight, divergence, and inaccuracies (Susnjak, 2022). Hence, AI-based chatbots are not working as intelligently as people expect. For instance, a recent report based on FB project M presented by Griffith, (2018) showed that 70% of the interactions between AI and humans failed. Another piece of information submitted by Forbes showed that approximately 80% of the customers that use e-commerce websites are reluctant to communicate with AI chatbots since they cannot perceive their actual needs (Forbes., 2021).
High error susceptibility
Personal virtual assistants (PVAs) are recently gaining significant popularity and becoming a prominent feature of mobile devices. Contrary to its growing use in the private context, the persistent implementation of PVAs at the organizational level has experienced widespread employee resistance. The execution of a specific task by using PVA on a smartphone device is quite simple but somewhat complex at the corporate level (Adam et al., 2021). The employees in an organization can rarely act on their own as there is a kind of interdepending environment within an organization, and the failure of PVA can severely impact the organization at many levels (e.g., financial loss). Moreover, the fear of job loss is another significant factor in employee resistance to PVAs. The prospect of PVAs causing confidential data leakage is one of the fundamental reasons for mistrust among individuals working in large organizations. Hornung and Smolnik (Hornung & Smolnik, 2022). designed a framework to study the role of artificial intelligence in invading the workplace and found the presence of negative emotions and dissatisfaction among employees regarding using PVAs at an organizational level.
Privacy and security concerns
At the individual level, the adverse effects of artificial intelligence are generally reflected in exacerbating privacy concerns. AI has the potential to get deep insights into the lives of users, thereby significantly inducing significant privacy risks. The electronic marketing industry uses AI for content and product recommendations (Grewal et al., 2021). Dickson, (2019) reported that AI-based voice assistant like Alexa has the potential to predict the status of an ongoing relationship between a consumer with a marketer by analyzing the tone of voice of the consumer. A recent study by Seo et al., (2022) reported that consumers are still hesitant to use voice assistants for online shopping due to privacy issues. AI-based facial recognition for online payments can induce numerous privacy risks because the human face can reveal much personal information like age, gender, and appearance. Moreover, online recommendations based on previous searches and personal experiences can eventually lead to privacy concerns and perceived information narrowing. Therefore, people are often reluctant to rely on these technologies (Li et al., 2021).
Promoting addictive behaviors
Due to its growing demand in the tech industry, the term "addictive" is observed as one of the greatest compliments given to an artifact. Due to the emerging paradigms based on AI-based technologies, the importance of research connected with addictive digital behaviors is also evolving. Current research focuses on exploring the approaches and methods used by tech companies that ultimately lead to addiction (Ferreri et al., 2018). The development of novel AI-based designs has been reported to be responsible for promoting addictive technology that is growing massively and becoming harder to resist. Moreover, AI and concomitant ethical debates bring novel perspectives on these addictive designs. AI-based algorithms have the potential to reshape the various aspects of human lives. With the development of novel algorithms capable of learning every user's experiences, habits, and schedules, addiction is expected to be attained at a comprehensive level along with sufficient granularity (Berthon et al., 2019). These addictive properties are generated by machine learning's ability to optimize the computable measures of engagement (Fourcade & Johns, 2020). According to a health standpoint, the excessive use of the internet, binge-watching of videos, obsessive use of social media, and problematic online game playing are some behaviors that can be assumed addictive (Burke et al., 2022). This so-called addiction induced by technology is now recognized as a proper health disorder (Vahia, 2013).
From an economic perspective, the growing need for these addictive technologies can be correlated with the new business models developed to capture and retain the user's attention (Bhargava & Velasquez, 2021). Diverse business companies and manufacturers widely employ AI-based algorithms to promote their services and various available products. For instance, these AI-based business strategies can be considered responsible for the exposure of adolescents and adults to content (either in the form of images, videos, or texts) related to pro-substance use, which has been further reported to be associated with high chances of substance use or substance addiction among those individuals (Burke et al., 2022). Indeed, addictive behaviors are becoming more common due to these addictive technologies. Furthermore, it would not be wrong to say that as our ability to detect addiction by using technology grows, so does our capability to substantially develop software and create experiences that progressively lead to addiction.
Promoting dark patterns
As these technologies are relatively emerging, different addictions are also being identified. The strategy used for gaining such user experiences includes using numerous sophisticated deceptive techniques to deliberately turn the knowledge collected from the users ultimately against them, also known as "dark patterns." These patterns are generally user interfaces intentionally developed by the designers to purposely manipulate or confuse the users by making it complicated for the users to demonstrate their actual preferences (Luguri & Strahilevitz, 2021; Waldman, 2020). For example, a video app called Tik Tok is developed to collect information about its users, including the content they watch, their caption, their location, the total time of use, and even the emotions that the video will develop. This bulk of data is consequently employed to do large-scale collaborative filtering and developing links that surpass human abilities, thereby giving a unique experience to each user that complements the neurodiversity of every individual user and makes it more addictive (Zhao, 2021). Famous social websites like YouTube, Facebook, Instagram, and others employ similar algorithms and AI-based engines. Mentioned above are some of the ways that are developed by artificial intelligence to make users more addicted. Although the harms caused by these AI-based designs on the users are apparent, tech companies continuously design such experiences to be more irresistible (Sin et al., 2022).
High Unemployment
Business organizations face significant challenges in successfully executing artificial intelligence strategies in their current business models. Moreover, they have failed to address the grave problem of how this technology will impact the human workforce. One of the most significant alarming concerns of AI is its potential to cause large-scale human unemployment globally, and this mass unemployment is not only expected within e-commerce, but as this technology is rapidly evolving, this could enormously impact global labor markets and eliminate jobs in all walks of life (Danaher, 2019). Though this argument might sound satirical, it is relatively instructive in the sense that it illustrates the idea of how the rise of AI can have an impact on the human world.
Conclusion
Artificial Intelligence has been around for many decades, but it has been evolving rapidly and recently sparking primary interest among business enterprises, widely becoming one of the hottest trends of 2023. As with every emerging technology, AI is also a source of excitement and skepticism. Artificial intelligence has several challenges, and people are expressing their anxiety and dissatisfaction over the prospects of AI wreaking havoc in the lives of humans. Undoubtedly, the impact of artificial intelligence on the global industry is irrefutable, and the most significant role humans can play in this perplexing situation is to ensure that this technology doesn't get out of hand. There is a dire need to improve artificial intelligence literacy and upskill to get the most out of it. Moreover, there is a need for more research to overcome numerous challenges before we can admit the immense transformational potential of this emerging technology.
Declaration
None
Ethical Approval
Not applicable
Competing interests
None
Authors' contributions
Mr. Stevie wrote the main body of the article, while Ms. Ammara added data regarding cons of Al and made figures.
Funding
None
Availability of data and materials
None
References
- Adam, M., Wessel, M., & Benlian, A. (2021). A1-based chatbots in customer service and their effects on user compliance. Electronic Markets, 31(2), 427-445.
- [Record #90 is using a reference type undefined in this output style.]
- Berthon, P., Pitt, L., & Campbell, C. (2019). Addictive de-vices: A public policy analysis of sources and solutions to digital addiction. Journal of Public Policy & Marketing, 38(4), 451-468.
- Bhargava, V. R., & Velasquez, M. (2021). Ethics of the attention economy: The problem of social media addiction. Business Ethics Quarterly, 31(3), 321-359.
- Bhutoria, A. (2022). Personalized education and artificial intelligence in the United States, China, and India: A systematic review using a human-in-the-loop model. Computers and Education: Artificial Intelligence, 3, 100068.
- Burke, S. A., Mahoney, A., Akhtar, A., & Hammer, A. (2022). Public Perspective on the Negative Impacts of Substance Use-Related Social Media Content on Adolescents: A Survey. Open Journal of Psychology, 77-83.
- Castagno, S., & Khalifa, M. (2020). Perceptions of artificial intelligence among healthcare staff: a qualitative survey study. Frontiers in artificial intelligence, 3, 578983.
- Cheng, X., Lin, X., Shen, X.-L., Zarifis, A., & Mou, J. . ((2022)). The dark sides of AI. Electronic Markets. 32((1)), 11-15.
- Chi, O. H., Denton, G., & Gursoy, D. (2020). Artificially intelligent device use in service delivery: A systematic review, synthesis, and research agenda. Journal of Hospitality Marketing & Management, 29(7), 757-786.
- Culkin, R., & Das, S. R. (2017). Machine learning in finance: the case of deep learning for option pricing. Journal of Investment Management, 15(4), 92-100.
- Curchoe, C. L., & Bormann, C. L. (2019). Artificial intelligence and machine learning for human reproduction and embryology presented at ASRM and ESHRE 2018. Journal of assisted reproduction and genetics, 36, 591-600.
- Danaher, J. (2019). The rise of the robots and the crisis of moral patiency. ai & Society, 34(1), 129-136.
- Dickson, E. (2019). Can Alexa and Facebook predict the end of your relationship. Retrieved October, 1, 2021.
- Dwivedi, Y. K., Hughes, L., Ismagilova, E., Aarts, G., Coombs, C., Crick, T., Duan, Y., Dwivedi, R., Edwards, J., & Eirug, A. (2021). Artificial Intelligence (AI): Multidisciplinary perspectives on emerging challenges, opportunities, and agenda for research, practice and policy. International Journal of Information Management, 57, 101994.
- Ferreri, F., Bourla, A., Mouchabac, S., & Karila, L. (2018). e-Addictology: an overview of new technologies for assessing and intervening in addictive behaviors. Frontiers in Psychiatry, 51.
- Floridi, L., Cowls, J., Beltrametti, M., Chatila, R., Chazerand, P., Dignum, V., Luetge, C., Madelin, R., Pagallo, U., & Rossi, F. (2021). An ethical framework for a good AI society: Opportunities, risks, principles, and recommendations. Ethics, governance, and policies in artificial intelligence, 19-39.
- Forbes. (2021). AI stats news: chatbots increase sales by 67% but 87% of consumers prefer humans.
- Fourcade, M., & Johns, F. (2020). Loops, ladders and links: the recursivity of social and machine learning. Theory and society, 49, 803-832.
- Gibney, E. (2022). How to shrink AI’s ballooning carbon footprint. Nature, 607(7920), 648-648.
- Goasduff, L. ( 2021). While advances in machine learning, computer vision, chatbots and edge artificial intelligence (AI) drive adoption, it's these trends that dominate this year’s Hype Cycle. .
- [Record #105 is using a reference type undefined in this output style.]
- Griffith, E., Simonite, . (2018). Facebook’s virtual assistant M is dead. So are chatbots. . Wired Business, 8.
- Hornung, O., & Smolnik, S. (2022). AI invading the workplace: negative emotions towards the organizational use of personal virtual assistants. Electronic Markets, 1-16.
- Li, J., Zhao, H., Hussain, S., Ming, J., & Wu, J. (2021). The dark side of personalization recommendation in short-form video applications: an integrated model from information perspective. International Conference on Information,
- Li, J. J., Bonn, M. A., & Ye, B. H. (2019). Hotel employee's artificial intelligence and robotics awareness and its impact on turnover intention: The moderating roles of perceived organizational support and competitive psychological climate. Tourism Management, 73, 172-181.
- Luguri, J., & Strahilevitz, L. J. (2021). Shining a light on dark patterns. Journal of Legal Analysis, 13(1), 43-109.
- Luo, X., Tong, S., Fang, Z., & Qu, Z. (2019). Frontiers: Machines vs. humans: The impact of artificial intelligence chatbot disclosure on customer purchases. Marketing Science, 38(6), 937-947.
- Ploug, T., & Holm, S. (2020). The four dimensions of contestable AI diagnostics-A patient-centric approach to explainable AI. Artificial Intelligence in Medicine, 107, 101901.
- Radhakrishnan, J., & Chattopadhyay, M. (2020). Determinants and barriers of artificial intelligence adoption–A literature review. Re-imagining Diffusion and Adoption of Information Technology and Systems: A Continuing Conversation: IFIP WG 8.6 International Conference on Transfer and Diffusion of IT, TDIT 2020, Tiruchirappalli, India, December 18–19, 2020, Proceedings, Part I,
- Seo, J., Lee, D., & Park, I. (2022). Can Voice Reviews Enhance Trust in Voice Shopping? The Effects of Voice Reviews on Trust and Purchase Intention in Voice Shopping. Applied Sciences, 12(20), 10674.
- Sin, R., Harris, T., Nilsson, S., & Beck, T. (2022). Dark patterns in online shopping: do they work and can nudges help mitigate impulse buying? Behavioural Public Policy, 1-27.
- Strubell, E., Ganesh, A., & McCallum, A. (2019). Energy and policy considerations for deep learning in NLP. arXiv preprint arXiv:1906.02243.
- Susnjak, T. (2022). ChatGPT: The end of online exam integrity? arXiv preprint arXiv:2212.09292.
- Tamburrini, G. (2022). The AI carbon footprint and responsibilities of AI scientists. Philosophies, 7(1), 4.
- Vahia, V. N. (2013). Diagnostic and statistical manual of mental disorders 5: A quick glance. Indian journal of psychiatry, 55(3), 220.
- Waldman, A. E. (2020). Cognitive biases, dark patterns, and the ‘privacy paradox’. Current opinion in psychology, 31, 105-109.
- Xu, Y., Liu, X., Cao, X., Huang, C., Liu, E., Qian, S., Liu, X., Wu, Y., Dong, F., & Qiu, C.-W. (2021). Artificial intelligence: A powerful paradigm for scientific research. The Innovation, 2(4).
- Zhao, Z. (2021). Analysis on the “Douyin (Tiktok) Mania” phenomenon based on recommendation algorithms. E3S Web of Conferences,